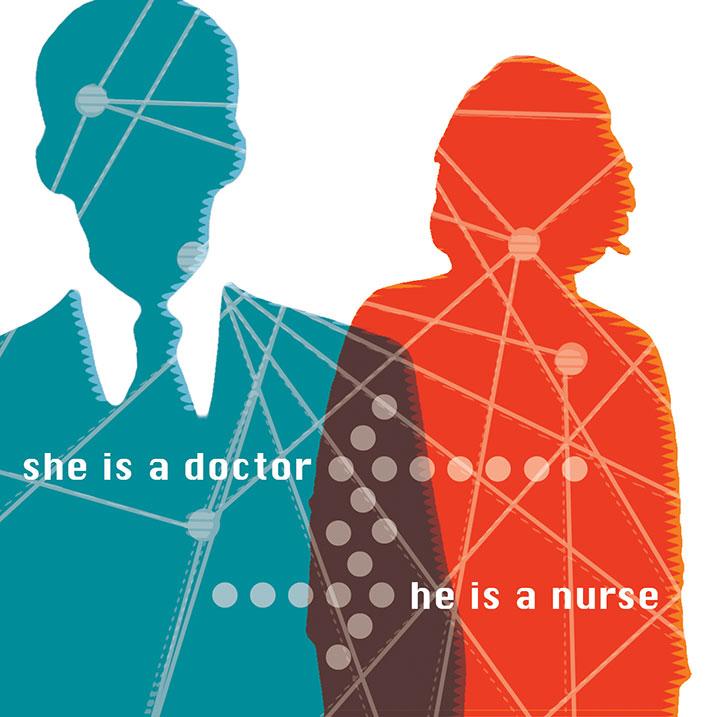
For the study, the researchers used a word-association tool called the implicit association test (IAT). For nearly two decades, psychologists have measured implicit biases with IAT, a method in which people are presented with two different categories — for instance, African American and European American, or pleasant and unpleasant — and are then asked to sort names, words, or photos that appear into each category in rapid succession. The results of these tests have been used to demonstrate a variety of biases, including that most Americans have an implicit preference for white versus black faces, young versus old people, thin versus fat bodies, and straight versus gay people, based on the words and images they associate with positive categories.
The Princeton researchers designed a similar test to run on a large body of pre-existing text drawn from across the web to reveal whether biases appeared in the language. They developed a word-embedding association test (WEAT) to measure how closely different words found online were associated with one another based on whether those words were used in similar contexts near the same kinds of language elsewhere online. When they ran their test, the researchers found that the associations between different words online mirrored eight common IAT results, including a preference for European American names versus African American names, and an association of math and science with males and the arts with females.“Many people have the common misconception that machines might be neutral or objective, but that is not the case because humans are teaching the machines,” says Caliskan, a fellow and postdoctoral research associate at the Center for Information Technology and a lecturer in computer science.
Having replicated several human biases with WEAT, the researchers then tested whether they could also reproduce statistics from the data. For instance, they looked up the percentage of women and men employed in different occupations and found that the degree of association between each of those professions and male and female words in their test sample of online text was very closely correlated to how male- or female-dominated each profession actually was.
“It’s astonishing how accurately these models are able to capture the world — the human biases and also statistical facts,” Caliskan says.
Joanna Bryson, a co-author of the paper and a professor at the University of Bath who was a visiting professor in 2015-16 at Princeton, says the results have important implications for people working in AI and for how we understand the role of language in passing on prejudices.
“Parts of our brains may just be picking up these biases directly from the language we’re exposed to, and other parts of our brains are consciously choosing what beliefs and biases to accept and reject,” Bryson says. She’s interested in trying to extend the work by applying WEAT to foreign languages to see if the associations vary, depending on different cultures.
“Some people think AI should be better than human intelligence,” Bryson adds. “Our work shows some of the reasons that that can’t be — because it’s bounded by us.”