Computer Science Researchers Call Out AI Hype as ‘Snake Oil’
Their critique of AI employment hiring tools won them a spot on Time magazine’s “Most Influential People in AI” list
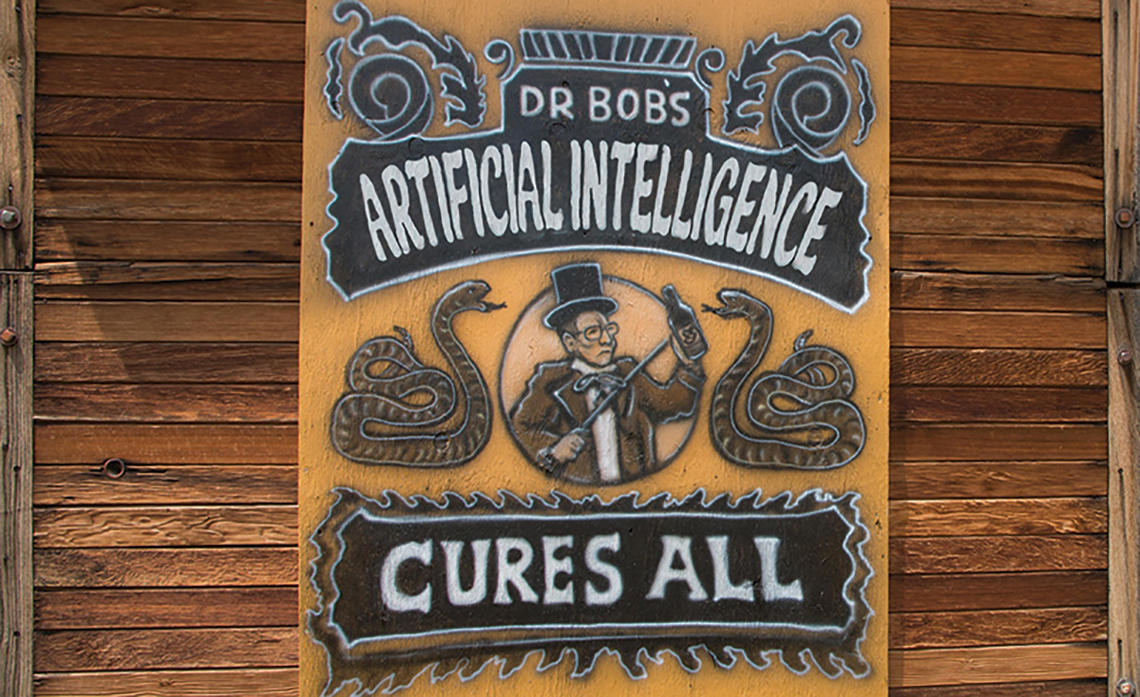
Companies receive hundreds, if not thousands, of job applications for open positions. So traditional ways of screening and interviewing candidates are breaking down, says Arvind Narayanan, a professor of computer science at Princeton. Desperate for solutions, businesses are turning to predictive AI tools, which promise to forecast candidate fit faster and more efficiently. But there’s a problem. “There’s a huge gap between how these technologies have been sold and what they can actually do,” says Narayanan, who is also director of Princeton’s Center for Information Technology Policy.
Narayanan and Sayash Kapoor, a third-year doctoral student at Princeton, have been calling out the predictive AI hype as “snake oil.” Their work won them inclusion in the 2023 Time100 “Most Influential People in AI” list. It’s also the topic of a book the duo co-authored, titled AI Snake Oil, expected to be published in 2024.
AI hype is something that companies recognize but keep perpetuating, Narayanan says. Part of the reason is because “AI tools, touted as math-based, efficient, and unbiased, have a veneer of authority companies are looking for,” he adds.
As assurance, companies sometimes use humans in the loop as arbiters over AI-based decisions. These humans are supposed to act as overseers of the AI-aided process but easily succumb to automation bias, Kapoor says. Too often these overseers don’t push back against the algorithm’s recommendations. “Often these humans are just showpieces in the decision-making loop, they’re just there to justify the company’s adoption of the tool, but they don’t have any real power on pushing back against automated decisions,” he says.
Errant predictive AI decisions — from unjust incarcerations to denial of acutely needed loans or jobs — can have enormous negative consequences. What can companies do better when using AI tools? Inquiring about the tool’s category — Is it generative or predictive AI? — is a great broad brushstroke question to begin with, Kapoor says. Generative AI, the kind used to create content, is based on actual technology advancements, and is less plagued by hype, he points out.
Companies should also ask about the data used to train the model. In the hiring process, for example, they want to avoid screening tools that ask all kinds of absurd and “sketchy” questions like “is your desk neat or untidy” as a predictor for job performance. The workaround is to create a bespoke tool with specific and relevant data from the organization’s own records, Kapoor says.
“With predictive AI, the shocking thing to me is that there has been virtually no improvement in the last 100 years. We’re using statistical formulas that were known when statisticians invented regression. That is still what is being used but is sold as something else.”
— Arvind Narayanan
Professor of computer science
Despite the frenetic pace of AI adoption, Narayanan does not believe it’s too late to “start making changes.” He advises companies to dig deeper and fix the underlying problem that’s making the adoption of imperfect tools a tempting proposition.
Breaking down the broad field of predictive AI into narrower subsets such as health care and hiring might help us tabulate progress. Use laws already on the books — the anti-discrimination law in hiring, for example — to effect change, he advises. Better funding of enforcement agencies will also help, says Kapoor.
While there has been some clamor for the private sector to self-police its AI policies, is it a matter of placing the fox in charge of the chicken coop? Narayanan says that toxic or biased AI outcomes can sully an enterprise’s reputation, so companies are trying to fix these aspects of data challenges. On the other hand, concerns about use of copyrighted data to create AI tools might not be a high priority. “In such cases, unless there’s legislation or successful lawsuits challenging business practices, nothing is going to change,” Narayanan cautions.
Narayanan is not very optimistic that predictive AI algorithms will get better with time. “With predictive AI, the shocking thing to me is that there has been virtually no improvement in the last 100 years. We’re using statistical formulas that were known when statisticians invented regression,” Narayanan says. “That is still what is being used but is sold as something else.”
0 Responses