Growing up in Iran, Amir Ali Ahmadi was singularly focused on one thing: tennis.
“I played seven hours a day for many, many years,” says Ahmadi, noting he played on Iran’s national junior tennis team at the age of 17 and dreamed of going pro.
When Ahmadi came to the United States for college, however, he quickly realized the likelihood of a tennis career was low. But he also noted that he was more advanced in math than his American peers. His intense focus switched to academics.
“I liked all areas of math, but I tended more towards the mathematics of algorithms and computer science,” says Ahmadi, who earned his bachelor’s degree in mathematics from the University of Maryland and his Ph.D. in electrical engineering and computer science from MIT. It was at MIT that he began specializing in the field of optimization.
“What draws me to optimization primarily is the universality of it,” he says. “Once you abstract this way of thinking about the universe, you can see everything as an optimization problem.”
Quick Facts
Title
Professor of Operations Research and Financial Engineering
Time at Princeton
9 years
Recent Class
Computing and Optimization for the Physical and Social Sciences
Ahmadi’s research
A Sampling
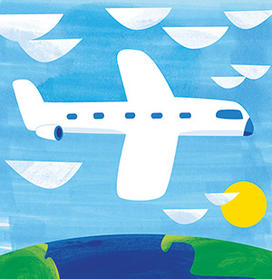
Doing More With Less
Ahmadi is interested in improving the efficiency and reliability of dynamical systems — mathematical models that describe objects moving through space and time, such as planets, airplanes, drones, and even diseases — especially when data is limited. For example, Ahmadi says, imagine a flying airplane suddenly experiences engine failure or wing damage. “The computer needs to … gather some information and quickly learn from it so that it can autonomously land the plane before it crashes.” In two different 2023 papers, Ahmadi and his colleagues showed how the behavior of dynamical systems can be learned from only a few of their standard trajectories, allowing the algorithms that apply to them to respond quickly even when new information is limited.
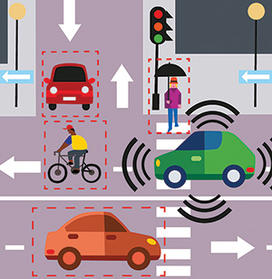
Providing More Control
Ahmadi also applies his optimization background to improve robots’ collision avoidance and stability. For example, if you want an autonomous car to drive from point A to point B, you need to program it to avoid obstacles, Ahmadi says. “How do you design a controller that will take you in the fastest time from A to B while making sure that there is no collision?” By increasing stability, or a robot’s ability to adjust to its environment to stay on track, you can make sure a land robot can account for a stone that might cause it to fall over or keep a flying drone on course even in strong wind.
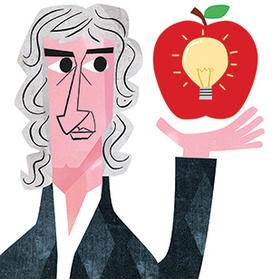
Optimizing Theory Itself
“I do a lot of work just in the pure theory of optimization,” Ahmadi says. For example, let’s say you want to invest Princeton’s endowment to maximize its return in one year without incurring too much risk — all in a socially responsible way. Perhaps the most widespread algorithm used for these kinds of processes is Newton’s method, originally developed by Isaac Newton in the 1600s. It involves using quadratic mathematical models to approximate solutions to problems that would be too difficult to solve precisely, even on a computer. In a paper published in November, Ahmadi and two graduate students show that new, more modern methods —specifically, using higher-order models, not just quadratic ones — can fine-tune Newton’s method to produce more accurate metrics in a shorter time. “In a nutshell, it’s about improving optimization itself,” he says.
0 Responses