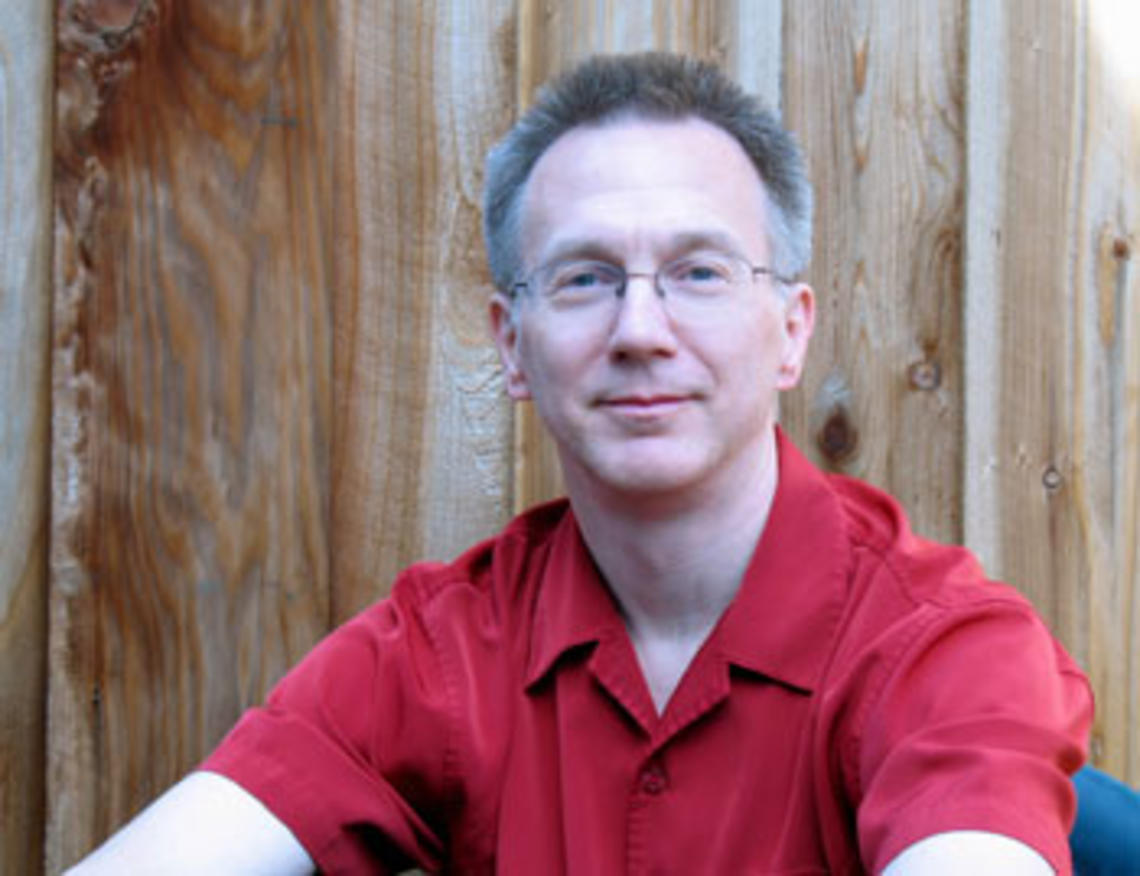
The book: The proliferation of “Big Data” and statistical analysis has been a boon for the sciences. However, as Judea Pearl and Dana Mackenzie *83 note in their new book, The Book of Why: The New Science of Cause and Effect (Basic Books), data or statistics on their own, without an understanding of their causes, makes it impossible to use them effectively. A rooster crows when the sun goes up with high statistical significance, but if you assume that the rooster causes the sunrise, your actual understanding of the world will be worse off.
Humans are quite good at deriving causal connections, but there remain fields where human intuition is not enough to chart the links between causes and effects. The Book of Why investigates the science of “causal inference,” developed in the past 30 years or so to provide a logically and scientifically rigorous framework for devising causation. Through causal inference, the authors show how it is possible not only to deduce why something happened, but why something did not happen, and most importantly, what would happen if you did something else — a speculative question not normally answerable without physical testing and an extremely important parameter for intelligent machines and AI.
The authors: Dana Mackenzie *83 is an award-winning science writer and the author of The Big Splat, or How Our Moon Came to Be.
Judea Pearl is a professor of computer science and statistics at UCLA, the winner of the 2011 Turing Award, and the author of three classic technical books on causality.
The new science does not have a fancy name: I call it simply “causal inference,” as do many of my colleagues. Nor is it particularly high-tech. The ideal technology that causal inference strives to emulate resides within our own minds. Some tens of thousands of years ago, humans began to realize that certain things cause other things and that tinkering with the former can change the latter. No other species grasps this, certainly not to the extent that we do. From this discovery came organized societies, then towns and cities, and eventually the science- and technology-based civilization we enjoy today. All because we asked a simple question: Why?
Causal inference is all about taking this question seriously. It posits that the human brain is the most advanced tool ever devised for managing causes and effects. Our brains store an incredible amount of causal knowledge which, supplemented by data, we could harness to answer some of the most pressing questions of our time. More ambitiously, once we really understand the logic behind causal thinking, we could emulate it on modern computers and create an “artificial scientist.” This smart robot would discover yet unknown phenomena, find explanations to pending scientific dilemmas, design new experiments, and continually extract more causal knowledge from the environment.
But before we can venture to speculate on such futuristic developments, it is important to understand the achievements that causal inference has tallied thus far. We will explore the way that it has transformed the thinking of scientists in almost every data-informed discipline and how it is about to change our lives. The new science addresses seemingly straightforward questions like these:
• How effective is a given treatment in preventing a disease?
• Did the new tax law cause our sales to go up, or was it our advertising campaign?
• What is the health-care cost attributable to obesity?
• Can hiring records prove an employer is guilty of a policy of sex discrimination?
• I’m about to quit my job. Should I?
These questions have in common a concern with cause-and-effect relationships, recognizable through words such as “preventing,” “cause,” “attributable to,” “policy,” and “should I.” Such words are common in everyday language, and our society constantly demands answers to such questions. Yet, until very recently, science gave us no means even to articulate, let alone answer, them.
By far the most important contribution of causal inference to mankind has been to turn this scientific neglect into a thing of the past. The new science has spawned a simple mathematical language to articulate causal relationships that we know as well as those we wish to find out about. The ability to express this information in mathematical form has unleashed a wealth of powerful and principled methods for combining our knowledge with data and answering causal questions like the five above.
Reviews: “Have you ever wondered about the puzzles of correlation and causation? This wonderful book has illuminating answers and it is fun to read.” – Daniel Kahneman, winner of the Nobel Memorial Prize in Economic Sciences
“The Book of Why not only delivers a valuable lesson on the history of ideas, but provides the conceptual tools needed to judge just what big data can and cannot deliver.” – The New York Times
0 Responses